Excluding Items Definition
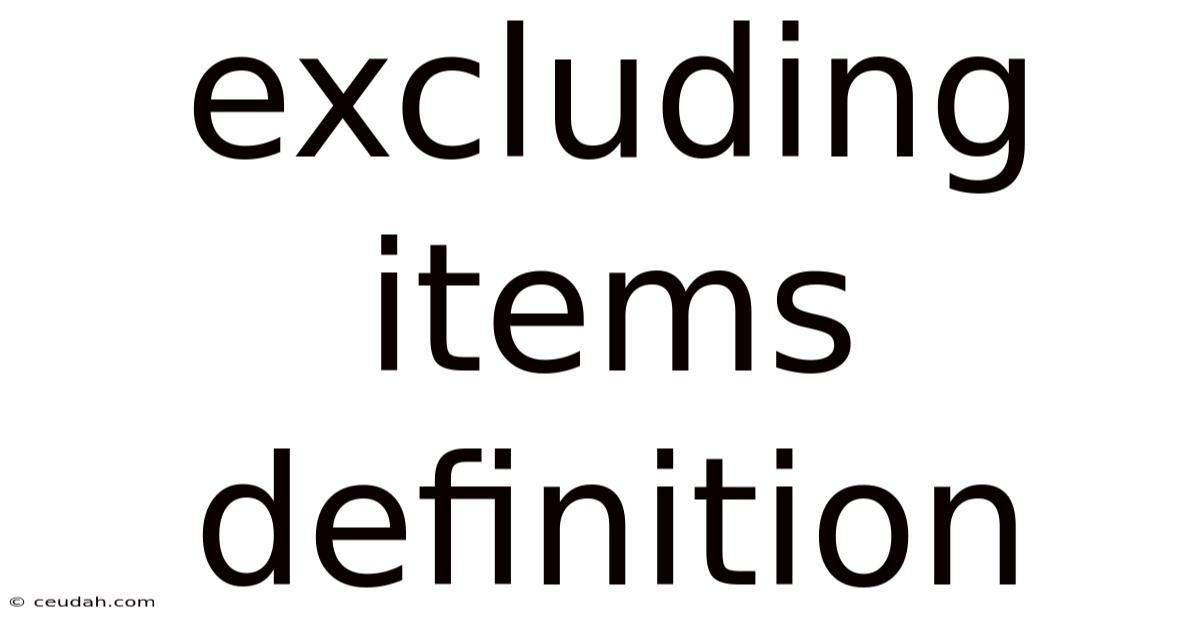
Discover more detailed and exciting information on our website. Click the link below to start your adventure: Visit Best Website meltwatermedia.ca. Don't miss out!
Table of Contents
Excluding Items: A Comprehensive Guide to Item Exclusion in Various Contexts
What makes item exclusion a critical consideration in today's data-driven world?
Item exclusion is a fundamental process impacting accuracy, efficiency, and decision-making across numerous fields.
Editor’s Note: This comprehensive guide to item exclusion has been published today.
Why Item Exclusion Matters
Item exclusion, the process of deliberately omitting specific items from a dataset, analysis, or calculation, is far more crucial than it initially appears. Its importance stems from its impact on the accuracy and reliability of results. Failing to exclude irrelevant or erroneous data can lead to flawed conclusions, impacting everything from scientific research and financial modeling to marketing campaigns and supply chain management. The application of item exclusion spans diverse sectors, including:
- Data Analysis & Statistics: Removing outliers or invalid data points is critical for accurate statistical analysis and preventing skewed results.
- Finance & Accounting: Excluding non-recurring items from financial statements ensures a clearer picture of underlying performance.
- E-commerce & Retail: Filtering out irrelevant products or customer segments optimizes marketing campaigns and inventory management.
- Supply Chain Management: Identifying and excluding faulty or obsolete items prevents disruptions and improves efficiency.
- Healthcare: Excluding patients with confounding factors ensures accurate clinical trial results and personalized treatment plans.
- Machine Learning: Removing noisy or irrelevant data enhances model accuracy and predictive power.
Overview of the Article
This article delves into the multifaceted world of item exclusion, exploring its diverse applications, methodologies, and implications. We will examine the reasons for exclusion, common techniques used, potential pitfalls to avoid, and best practices for ensuring accurate and reliable results. Readers will gain a thorough understanding of this critical process and its impact across various domains.
Research and Effort Behind the Insights
This article draws upon extensive research, incorporating examples from diverse fields, established statistical methods, and best practices from industry experts. The information presented is based on a thorough review of relevant literature, case studies, and practical applications of item exclusion techniques.
Key Takeaways
Key Aspect | Description |
---|---|
Reasons for Exclusion | Outliers, errors, irrelevant data, confounding factors, bias, etc. |
Exclusion Methods | Filtering, data cleaning, outlier detection techniques, statistical methods |
Potential Pitfalls | Incorrect application, bias introduction, data loss, misinterpretation of results |
Best Practices | Clear definition of inclusion/exclusion criteria, transparency, documentation, validation |
Impact on Decision-Making | Improved accuracy, enhanced efficiency, better resource allocation, informed strategic choices |
Let's dive deeper into the key aspects of item exclusion, starting with its foundational principles and real-world applications.
Exploring the Key Aspects of Item Exclusion
-
Identifying Items for Exclusion: The first and most critical step is identifying which items warrant exclusion. This requires a clear understanding of the research question, the dataset's characteristics, and potential sources of bias or error. Visual inspection of data, statistical outlier detection methods (e.g., box plots, Z-scores), and domain-specific knowledge are all essential tools.
-
Exclusion Methods and Techniques: Numerous methods exist for excluding items, each suited to different contexts. These include:
- Filtering: A simple but effective method involves setting criteria to automatically remove items that don't meet specific thresholds (e.g., removing data points outside a certain range).
- Data Cleaning: This broader process involves identifying and correcting errors, inconsistencies, and missing values. Items with irreparable errors may need exclusion.
- Outlier Detection: Advanced statistical methods like robust regression, k-nearest neighbors, and Isolation Forest can identify outliers that may unduly influence results.
- Imputation: Instead of outright exclusion, some approaches replace missing or erroneous values with estimated ones. This can be preferable when data loss is significant.
- Winsorization and Trimming: These techniques modify outliers rather than entirely removing them. Winsorization replaces extreme values with less extreme ones, while trimming removes a fixed percentage of the highest and lowest values.
-
Impact of Exclusion on Results: The choice of exclusion method significantly impacts the results. For instance, excluding outliers can increase the precision of statistical estimations but may also lead to a loss of information if the outliers represent a genuine phenomenon. Transparency about the exclusion criteria and their impact is crucial for the reproducibility and validity of findings.
-
Documentation and Transparency: Detailed documentation of the exclusion process is paramount. This includes specifying the criteria used, the methods applied, and the rationale behind each exclusion decision. This fosters reproducibility and allows others to scrutinize the methodology.
-
Validation and Verification: After applying item exclusion, it's essential to validate the results. This may involve comparing the results with those obtained using different exclusion methods or examining the impact of different exclusion thresholds. Cross-validation techniques are especially useful in this context.
Closing Insights
Item exclusion is a crucial step in ensuring the accuracy and reliability of data analysis and decision-making. The choice of methods and the criteria for exclusion must be carefully considered and documented. Transparency and validation are essential to maintain the integrity and reproducibility of results. Failing to properly address item exclusion can lead to flawed conclusions, misinformed decisions, and wasted resources. The responsible application of item exclusion is a cornerstone of sound data practice across all fields.
Exploring the Connection Between Outliers and Item Exclusion
Outliers, data points significantly deviating from the rest of the data, frequently necessitate exclusion. They can arise from measurement errors, data entry mistakes, or represent genuinely unusual events. Their presence can skew statistical analyses, distorting measures of central tendency (mean, median) and variability.
Roles and Real-World Examples:
- Finance: An unusually large transaction might represent fraud or an error needing exclusion from financial reporting.
- Healthcare: A patient with an atypical response to a treatment might be an outlier whose data is excluded from a clinical trial analysis to avoid skewing results.
- Manufacturing: An unusually high defect rate from a specific production run might indicate a problem requiring investigation; the data from that run might be excluded from overall quality assessments.
Risks and Mitigations:
- Risk: Incorrectly identifying and excluding outliers can lead to a loss of valuable information, especially if outliers represent genuine phenomena.
- Mitigation: Employ robust outlier detection methods, explore alternative analyses, and carefully document the rationale behind any exclusions.
Impact and Implications:
The impact of excluding outliers is significant. It can lead to more reliable statistical measures, improved model accuracy, and more informed decisions. However, it’s crucial to balance this with the potential loss of valuable information.
Further Analysis of Outliers
Outliers can stem from various sources:
- Measurement Error: Faulty equipment or human error can lead to inaccurate measurements.
- Data Entry Errors: Mistakes during data entry are a common source of outliers.
- Genuine Anomalies: Outliers may represent genuine events or phenomena, providing valuable insights.
Cause-and-Effect Relationships:
Outliers can influence the overall results by:
- Inflating the mean: Outliers pull the mean towards their extreme values, distorting the average.
- Increasing variability: Outliers increase the range and standard deviation, affecting measures of dispersion.
- Reducing the effectiveness of statistical models: Outliers can negatively affect the performance of regression models and other statistical methods.
Significance and Applications:
Understanding and addressing outliers is crucial for:
- Improving data quality: Cleaning data and removing errors is a fundamental step in data analysis.
- Enhancing model accuracy: Removing outliers can improve the accuracy and robustness of predictive models.
- Facilitating more reliable decision-making: Reliable data leads to more informed and effective decisions.
FAQ Section
-
Q: What are the ethical considerations of excluding items? A: Transparency and justification are vital. The rationale for exclusion must be clearly documented to avoid accusations of bias or manipulation.
-
Q: How do I choose the right exclusion method? A: The optimal method depends on the context, the nature of the data, and the research question. Consider the potential impact of different methods on the results.
-
Q: Can I exclude too many items? A: Yes, over-excluding items can lead to a loss of valuable information and biased results. A balance must be struck between removing problematic data and preserving the richness of the dataset.
-
Q: What if I'm unsure whether an item should be excluded? A: Consult with domain experts, conduct sensitivity analyses, and carefully document your considerations.
-
Q: How do I handle missing data? A: Strategies include imputation (replacing missing values with estimates), exclusion (removing items with missing data), or using analytical methods robust to missing data.
-
Q: What software can help with item exclusion? A: Various statistical software packages (R, SPSS, SAS) and data analysis tools (Python libraries like Pandas and Scikit-learn) provide functionality for data cleaning, outlier detection, and filtering.
Practical Tips
-
Define clear inclusion/exclusion criteria: Establish specific rules to determine which items should be included or excluded before starting the analysis.
-
Use visual inspection: Explore your data visually (e.g., histograms, scatter plots) to identify potential outliers or errors.
-
Employ statistical methods: Utilize outlier detection techniques to identify data points significantly deviating from the norm.
-
Document your methods and rationale: Maintain a clear record of the decisions made, the reasons behind them, and the chosen methods.
-
Validate your results: Compare your findings with alternative analyses or sensitivity analyses to check the robustness of your conclusions.
-
Consult with experts: Seek advice from domain experts to ensure that the exclusion criteria are appropriate and relevant.
-
Use appropriate software: Utilize statistical software or data analysis tools to streamline the item exclusion process.
-
Be transparent: Clearly communicate your item exclusion process and its impact on the results in any reports or publications.
Final Conclusion
Item exclusion, though often overlooked, is a critical process impacting the accuracy and reliability of data analysis across numerous fields. Understanding the various reasons for exclusion, employing appropriate methods, and maintaining transparency are crucial for ensuring the integrity of research and decision-making. By adopting best practices and carefully considering the implications of exclusion, researchers and analysts can enhance the quality of their work and generate more reliable and insightful results. The thoughtful application of item exclusion is not merely a technical step but a fundamental aspect of rigorous and ethical data handling.
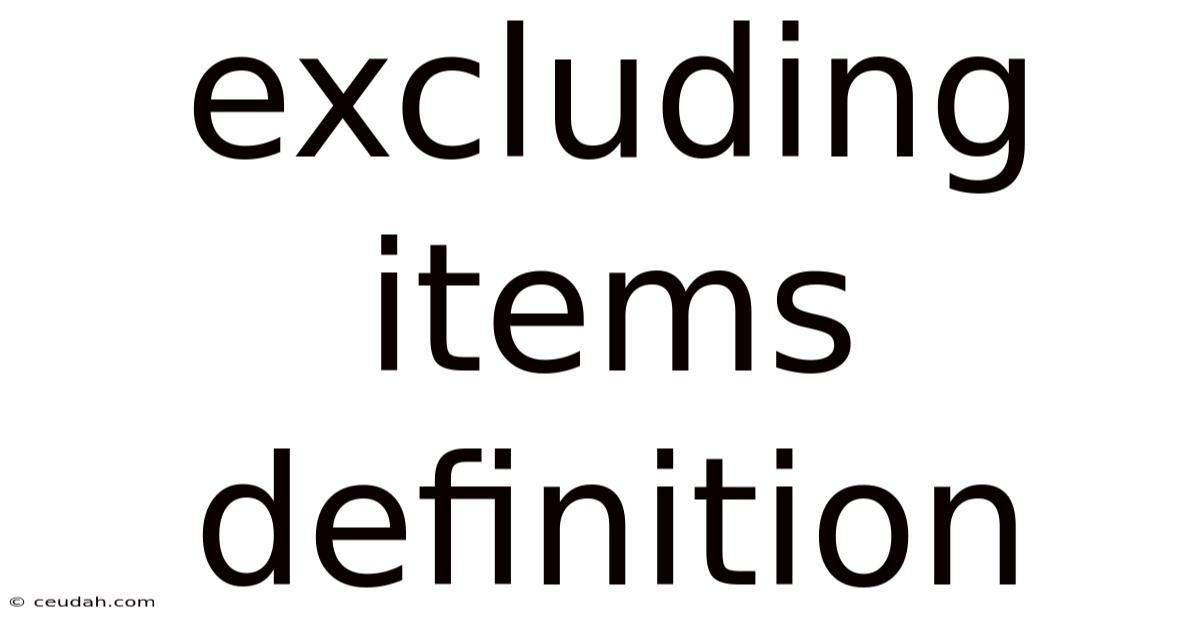
Thank you for visiting our website wich cover about Excluding Items Definition. We hope the information provided has been useful to you. Feel free to contact us if you have any questions or need further assistance. See you next time and dont miss to bookmark.
Also read the following articles
Article Title | Date |
---|---|
What Insurance Is Required When Financing A Car | Mar 26, 2025 |
How To Get Top Surgery Covered By Insurance | Mar 26, 2025 |
How Much Does A 72 Hour Hold Cost Without Insurance | Mar 26, 2025 |
How Long Do You Have To Add A New Driver To Your Insurance Policy | Mar 26, 2025 |
Ex Distribution Definition | Mar 26, 2025 |