Predictive Analytics Definition Model Types And Uses
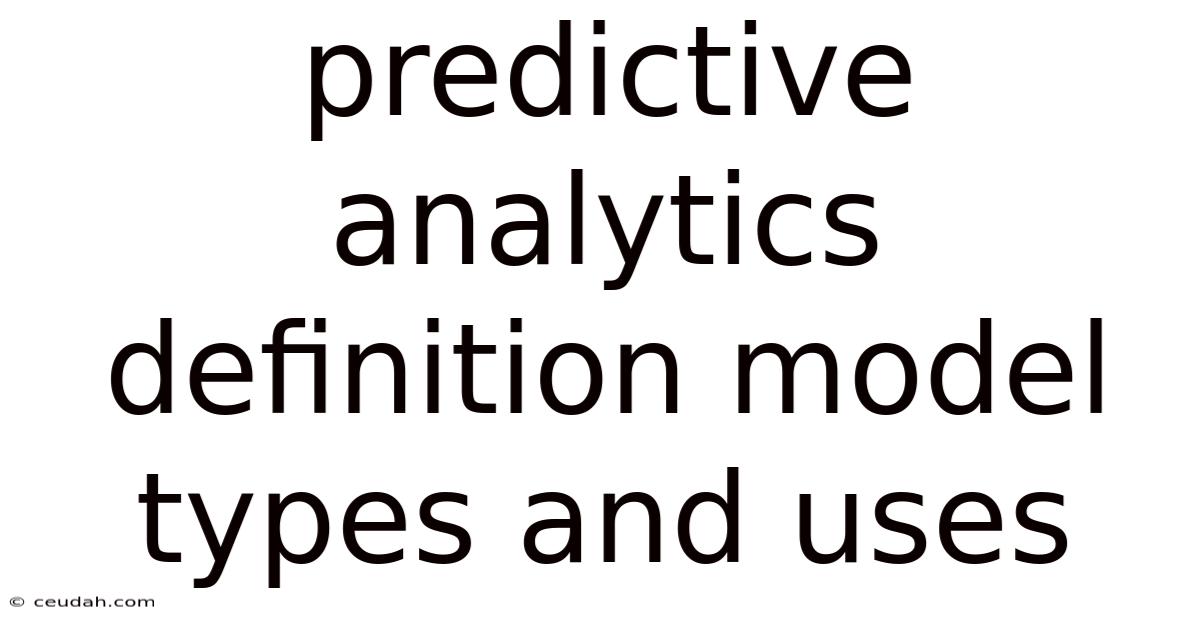
Discover more detailed and exciting information on our website. Click the link below to start your adventure: Visit Best Website meltwatermedia.ca. Don't miss out!
Table of Contents
Predictive Analytics: Unveiling the Future Through Data
What makes predictive analytics a game-changer in today’s landscape?
Predictive analytics is revolutionizing decision-making across industries, offering unprecedented insights and driving data-driven strategies for success.
Editor’s Note: Predictive analytics has been published today.
Why Predictive Analytics Matters
In today's data-saturated world, the ability to anticipate future outcomes is no longer a luxury but a necessity. Predictive analytics, a powerful subset of data analytics, empowers organizations to do just that. By leveraging historical data, statistical algorithms, and machine learning techniques, predictive analytics transforms raw data into actionable insights, enabling proactive decision-making and strategic planning. Its importance stems from its capacity to improve efficiency, reduce risks, and unlock significant opportunities across diverse sectors, from healthcare and finance to marketing and manufacturing. Predictive models help businesses optimize operations, personalize customer experiences, and gain a competitive edge in rapidly evolving markets. The ability to forecast demand, predict customer churn, or identify potential fraud significantly impacts profitability and long-term sustainability.
Overview of the Article
This article explores the multifaceted world of predictive analytics, encompassing its definition, various modeling techniques, and extensive applications across various industries. Readers will gain a comprehensive understanding of the core concepts, learn about different model types, and discover how these powerful tools are reshaping strategic decision-making. The article will also delve into the crucial connection between data quality and model accuracy, highlighting best practices for successful implementation.
Research and Effort Behind the Insights
This article draws upon extensive research, including peer-reviewed studies, industry reports from reputable sources like Gartner and Forrester, and case studies from leading organizations successfully employing predictive analytics. The insights presented are based on a rigorous analysis of existing literature and practical applications, ensuring accuracy and relevance.
Key Takeaways
Key Aspect | Description |
---|---|
Definition | Using data, statistical algorithms, and machine learning to predict future outcomes. |
Model Types | Regression, classification, time series, clustering, etc. |
Data Quality | Crucial for accurate and reliable predictions. |
Applications | Fraud detection, customer churn prediction, risk assessment, demand forecasting. |
Benefits | Improved decision-making, reduced risks, increased efficiency, competitive advantage. |
Challenges | Data limitations, model complexity, interpretation of results. |
Let’s dive deeper into the key aspects of predictive analytics, starting with its foundational principles and real-world applications.
Exploring the Key Aspects of Predictive Analytics
1. Defining Predictive Analytics:
Predictive analytics uses historical data, statistical algorithms, and machine learning techniques to identify the likelihood of future outcomes. Unlike descriptive analytics, which focuses on understanding past events, predictive analytics aims to forecast future trends and behaviors. This forecasting is not about making absolute predictions but rather assigning probabilities to different potential scenarios. The accuracy of these predictions depends heavily on the quality and relevance of the data used, as well as the sophistication of the chosen analytical model.
2. Predictive Modeling Techniques:
Several modeling techniques fall under the umbrella of predictive analytics. The choice of model depends on the specific problem, the type of data available, and the desired outcome. Some common techniques include:
-
Regression Models: These models predict a continuous outcome variable (e.g., sales revenue, stock price). Linear regression, logistic regression, and polynomial regression are popular examples.
-
Classification Models: These models predict a categorical outcome variable (e.g., customer churn – yes/no, fraud – yes/no). Decision trees, support vector machines (SVMs), and naive Bayes are commonly used classification algorithms.
-
Time Series Models: These models predict future values based on past values of a variable over time (e.g., stock prices, weather patterns). ARIMA (Autoregressive Integrated Moving Average) and exponential smoothing are frequently employed.
-
Clustering Models: These models group similar data points together based on their characteristics (e.g., customer segmentation). K-means clustering and hierarchical clustering are common techniques.
-
Neural Networks: These complex models, inspired by the human brain, can handle highly non-linear relationships in data and are particularly effective for complex prediction tasks. Deep learning, a subfield of neural networks, is rapidly advancing predictive analytics capabilities.
3. Data Quality and Preprocessing:
The accuracy of predictive models heavily relies on the quality of the input data. Data preprocessing is a crucial step that involves cleaning, transforming, and preparing the data for analysis. This may include handling missing values, dealing with outliers, and converting data into a suitable format for the chosen model. Inaccurate, incomplete, or biased data will lead to unreliable and potentially misleading predictions.
4. Model Evaluation and Selection:
Once a model is built, it must be rigorously evaluated to assess its performance. Metrics like accuracy, precision, recall, and F1-score are commonly used to evaluate classification models, while metrics like R-squared and mean squared error are used for regression models. Model selection involves choosing the model that performs best based on these evaluation metrics and other considerations, such as interpretability and computational cost.
5. Implementation and Deployment:
After model selection, the model is deployed to make predictions on new data. This may involve integrating the model into existing systems or creating a new application. Regular monitoring and retraining of the model are crucial to maintain its accuracy and relevance over time, as data patterns can shift.
6. Ethical Considerations:
The use of predictive analytics raises ethical considerations, particularly regarding bias and fairness. Biases in the training data can lead to discriminatory outcomes, highlighting the need for careful data selection and model evaluation to ensure fairness and equity. Transparency and explainability are also important aspects to build trust and avoid unintended consequences.
Closing Insights
Predictive analytics is not merely a technological advancement; it’s a transformative force with far-reaching implications across industries. Its ability to forecast future trends, optimize resource allocation, and personalize customer experiences offers a significant competitive advantage. From enhancing healthcare through early disease detection to optimizing supply chains in manufacturing, its applications are vast and continually expanding. The responsible and ethical deployment of predictive analytics will be crucial in harnessing its full potential while mitigating potential risks.
Exploring the Connection Between Data Quality and Predictive Analytics
Data quality is inextricably linked to the accuracy and reliability of predictive analytics models. Poor data quality can lead to inaccurate predictions, misleading insights, and ultimately, flawed decision-making. The relationship can be summarized as follows:
Roles: Data acts as the raw material for predictive models. The quality of this material directly impacts the quality of the final product – the prediction.
Real-World Examples: A retail company using faulty sales data to predict future demand may overstock or understock products, leading to lost revenue or dissatisfied customers. A bank using inaccurate credit history data to assess loan applications may approve risky loans, increasing the risk of defaults.
Risks and Mitigations: Incomplete data, inconsistent data formats, and data entry errors are significant risks. Mitigations include data cleaning, validation, and standardization procedures, as well as implementing robust data governance frameworks.
Impact and Implications: Inaccurate predictions can lead to wasted resources, missed opportunities, reputational damage, and financial losses. High-quality data, on the other hand, enables accurate predictions, improves decision-making, and enhances overall business performance.
Further Analysis of Data Quality
The impact of data quality on predictive analytics can be further analyzed through a cause-and-effect lens:
Cause | Effect |
---|---|
Incomplete Data | Inaccurate predictions, biased models, limited scope of analysis. |
Inconsistent Data Formats | Difficulty in data integration and analysis, errors in data processing. |
Data Entry Errors | Inaccurate predictions, misleading insights, flawed decision-making. |
Outdated Data | Predictions may not reflect current trends and market conditions. |
Biased Data | Models may perpetuate existing biases, leading to unfair or discriminatory outcomes. |
FAQ Section
-
What is the difference between predictive and descriptive analytics? Descriptive analytics summarizes past data, while predictive analytics forecasts future outcomes.
-
What types of businesses benefit most from predictive analytics? Almost any business can benefit, but those with large datasets and a need for proactive decision-making will see the greatest returns.
-
How much does predictive analytics cost? Costs vary widely depending on the complexity of the project, the size of the dataset, and the expertise required.
-
What are the ethical concerns surrounding predictive analytics? Bias in data can lead to unfair or discriminatory outcomes; transparency and explainability are crucial.
-
How can I improve the accuracy of my predictive models? Focus on data quality, experiment with different model types, and rigorously evaluate model performance.
-
What are some common challenges in implementing predictive analytics? Data limitations, model complexity, interpretation of results, and lack of skilled personnel.
Practical Tips
- Prioritize data quality: Invest in data cleaning, validation, and standardization procedures.
- Clearly define your objectives: What specific questions do you want to answer?
- Choose the right model: Select a model appropriate for your data and objectives.
- Rigorously evaluate your models: Use appropriate metrics to assess model performance.
- Continuously monitor and retrain your models: Data patterns change over time.
- Consider ethical implications: Ensure fairness and avoid bias in your models.
- Collaborate with data scientists: Seek expert help if needed.
- Start with a pilot project: Test your approach on a smaller scale before deploying it widely.
Final Conclusion
Predictive analytics is transforming how businesses operate and make decisions. By harnessing the power of data, organizations can gain a competitive edge, mitigate risks, and unlock new opportunities for growth. While challenges exist, the potential benefits of predictive analytics are undeniable. By understanding the core concepts, choosing the right models, and prioritizing data quality, organizations can unlock the transformative power of predictive analytics and shape a more data-driven future. Further exploration of specific applications within various industries will reveal even more compelling opportunities for innovation and strategic advantage.
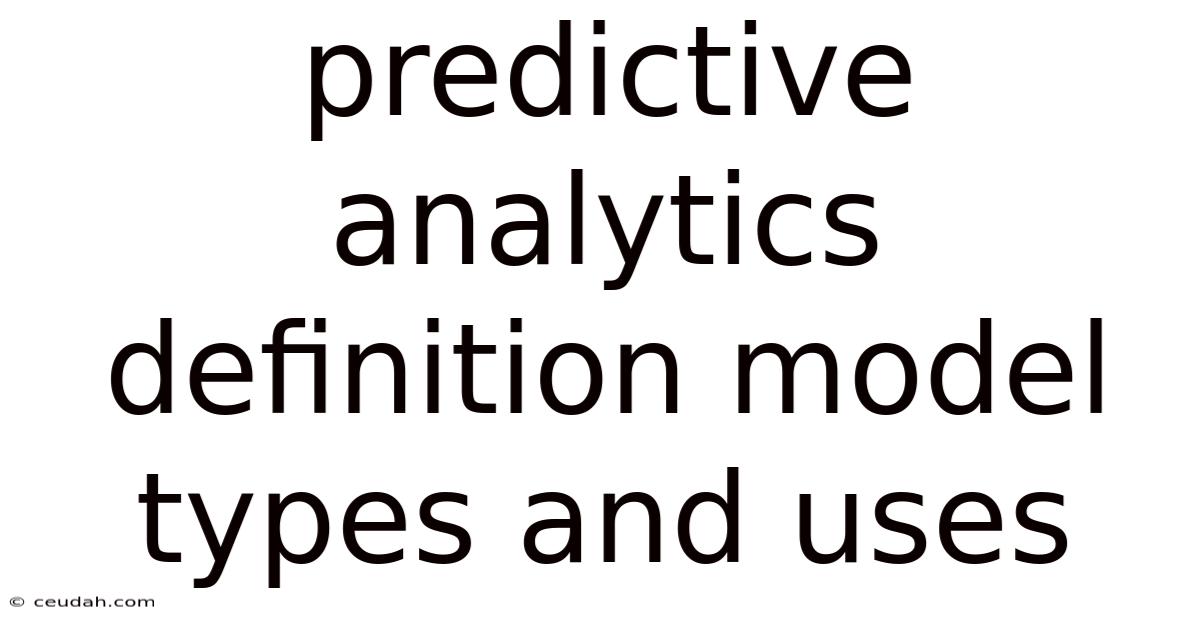
Thank you for visiting our website wich cover about Predictive Analytics Definition Model Types And Uses. We hope the information provided has been useful to you. Feel free to contact us if you have any questions or need further assistance. See you next time and dont miss to bookmark.
Also read the following articles
Article Title | Date |
---|---|
Profit Motive Definition Economic Theory Characteristics | Apr 15, 2025 |
How Many Years Can You Carry Forward Residential Energy Credit | Apr 15, 2025 |
How Do I Get An Apartment With Bad Credit | Apr 15, 2025 |
How Long Do Student Loans Stay On Your Credit After Paid Off | Apr 15, 2025 |
How Long Does It Take Chime To Give Provisional Credit | Apr 15, 2025 |