Probable Maximum Loss Definition And How To Calculate It
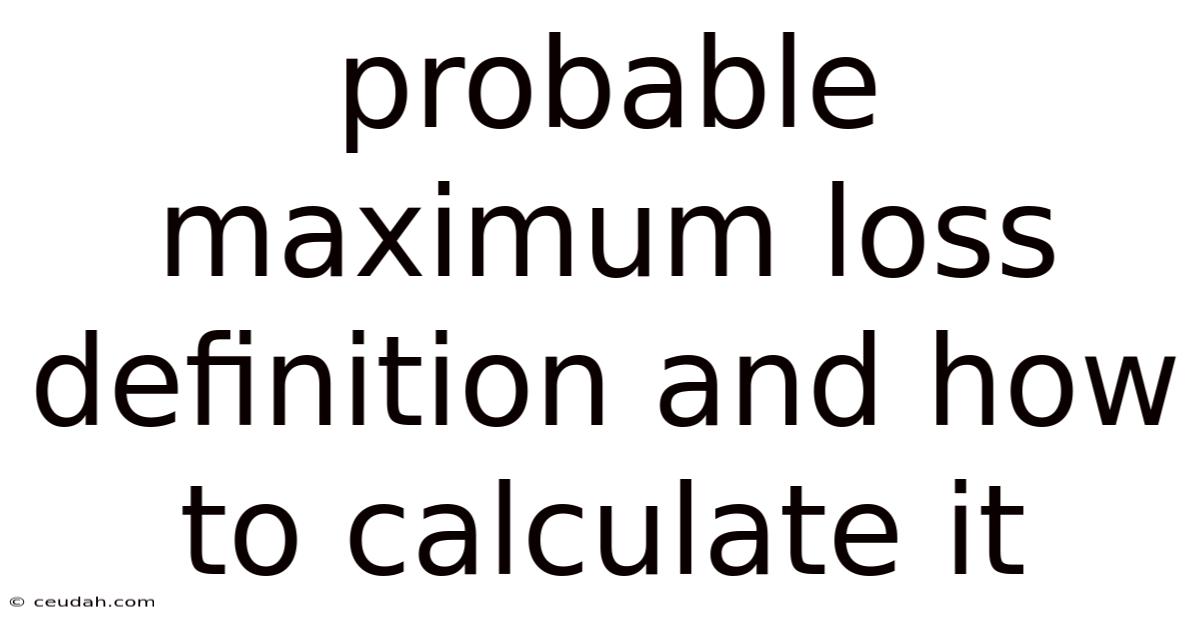
Discover more detailed and exciting information on our website. Click the link below to start your adventure: Visit Best Website meltwatermedia.ca. Don't miss out!
Table of Contents
Probable Maximum Loss (PML): Definition and Calculation Methods
What makes Probable Maximum Loss a crucial concept in risk management?
PML is a vital tool for insurers, reinsurers, and portfolio managers, enabling them to accurately assess and mitigate potential financial losses.
Editor’s Note: This article on Probable Maximum Loss (PML) has been published today, providing readers with the latest insights and calculation methods.
Why Probable Maximum Loss Matters
Understanding and accurately calculating Probable Maximum Loss (PML) is paramount for several key stakeholders. For insurance companies, PML is crucial for setting appropriate premiums, reserving capital, and managing their overall risk exposure. Reinsurance companies rely on PML calculations to assess the potential impact of catastrophic events on their portfolios and design effective reinsurance programs. Portfolio managers, especially those investing in catastrophe bonds or other weather-related securities, use PML estimations to make informed investment decisions and manage their risk profiles. Accurate PML calculations ultimately contribute to greater financial stability and resilience in the face of unpredictable events. The ability to quantify potential losses allows for better resource allocation, more effective risk mitigation strategies, and a more robust financial ecosystem. This is especially critical in industries like insurance and finance, where unforeseen events can have cascading consequences.
Overview of the Article
This article provides a comprehensive overview of Probable Maximum Loss (PML), exploring its definition, calculation methodologies, and practical applications. We will delve into different modeling techniques, discuss the key assumptions involved, and highlight the importance of accurate data input. Readers will gain a solid understanding of PML and its significance in managing various risks. The article will also examine the limitations of PML and emphasize the importance of considering other risk assessment tools in a holistic risk management framework.
Research and Effort Behind the Insights
The insights presented in this article are based on extensive research drawing upon industry reports, academic publications, and practical experience in risk modeling. We have reviewed numerous case studies and consulted with experts in actuarial science, catastrophe modeling, and risk management to ensure accuracy and practical relevance. The calculation methods described reflect current best practices and incorporate advancements in modeling techniques.
Key Takeaways
Key Aspect | Description |
---|---|
Definition of PML | The maximum loss an insurer or reinsurer could reasonably expect to experience from a single event. |
Calculation Methods | Various methods exist, including statistical analysis, Monte Carlo simulations, and deterministic models. |
Data Requirements | Accurate and comprehensive data on exposure, vulnerability, and hazard are crucial for accurate PML estimation. |
Limitations of PML | PML is a probabilistic estimate, and actual losses may vary. |
Importance of Holistic Risk Management | PML should be integrated into a broader risk management framework that considers other risk factors. |
Smooth Transition to Core Discussion
Let's delve into a detailed explanation of PML, starting with a precise definition and then exploring the intricacies of its calculation methods.
Exploring the Key Aspects of Probable Maximum Loss
-
Defining Probable Maximum Loss: PML represents the maximum probable loss an insurer or reinsurer could reasonably expect to suffer from a single event, such as a hurricane, earthquake, or fire. It’s not the absolute worst-case scenario, but rather a statistically derived estimate representing a high-likelihood, high-impact loss. The "probable" aspect acknowledges the inherent uncertainties associated with catastrophic events.
-
Data Requirements for PML Calculation: Accurate PML estimation hinges on the quality and completeness of the underlying data. This includes:
- Exposure Data: A detailed inventory of insured assets, their location, construction type, and value. This is often the most challenging aspect, requiring thorough data collection and verification.
- Vulnerability Data: Information about the susceptibility of assets to different types of hazards. This might involve engineering assessments, building codes, and historical damage data.
- Hazard Data: Probabilistic or deterministic data on the frequency and severity of various hazards. This often involves using catastrophe models that simulate the physical processes of natural hazards and their impact on exposed assets.
-
Calculation Methodologies: Several methods are used to calculate PML, each with its strengths and limitations:
- Statistical Methods: These methods analyze historical loss data to estimate the probability distribution of potential losses. They can be relatively simple to implement but require sufficient historical data, which might not always be available, especially for rare events.
- Monte Carlo Simulation: This sophisticated technique involves running numerous simulations, each with slightly different inputs, to generate a distribution of potential losses. It offers greater flexibility in handling uncertainty and can incorporate complex interactions between different factors.
- Deterministic Models: These models use pre-defined scenarios to estimate losses. While simpler than Monte Carlo simulations, they offer less flexibility and may not capture the full range of potential outcomes. They are often used for quick estimations or when data is limited.
-
Assumptions and Limitations: All PML calculations rely on several key assumptions, including:
- Model Accuracy: The accuracy of the chosen model and the underlying data significantly impacts the PML estimate.
- Data Completeness: Gaps in the data can lead to underestimation or overestimation of the PML.
- Correlation of Events: The possibility of multiple events occurring simultaneously is often not fully considered in simpler models.
- Changing Risk Landscape: PML estimations need to be regularly updated to reflect changes in exposure, vulnerability, and hazard characteristics.
-
Integrating PML into Holistic Risk Management: PML should not be viewed in isolation. It's one piece of a larger risk management puzzle. Other factors, such as operational risks, financial risks, and regulatory compliance, must be considered to create a comprehensive risk profile.
Exploring the Connection Between Catastrophe Modeling and PML
Catastrophe modeling plays a vital role in PML calculation. These sophisticated computer models simulate the physical processes of natural hazards (e.g., hurricanes, earthquakes, floods) and their impact on exposed assets. They incorporate a wide range of factors, including wind speed, rainfall intensity, ground motion, and building characteristics. By simulating thousands of potential events, catastrophe models generate a probability distribution of potential losses, which is then used to estimate the PML.
The accuracy of catastrophe modeling is directly tied to the quality of the input data and the sophistication of the model itself. Advanced models incorporate high-resolution geographical data, detailed building inventories, and probabilistic hazard assessments. However, even the most advanced models have limitations, and their outputs should be interpreted with caution.
Further Analysis of Catastrophe Modeling
Aspect of Catastrophe Modeling | Description |
---|---|
Input Data | High-resolution geographic data, building inventories, vulnerability curves, and hazard scenarios (e.g., earthquake magnitude, hurricane wind speed). |
Model Structure | Physical models simulate the propagation of hazards, while vulnerability models assess the damage to assets based on hazard intensity. |
Output Data | Probability distributions of losses at different return periods (e.g., 10-year, 50-year, 100-year events). |
Model Validation | Comparison with historical loss data to evaluate the accuracy of model predictions. |
Limitations | Uncertainties in hazard modeling, vulnerability assessment, and data availability can impact model accuracy. |
FAQ Section
-
Q: What is the difference between PML and Maximum Possible Loss (MPL)? A: PML represents the maximum loss that is reasonably likely to occur, while MPL represents the absolute worst-case scenario, regardless of its probability. PML is a more practical measure for risk management because it focuses on the losses that are statistically significant.
-
Q: How often should PML be recalculated? A: PML should be regularly updated, ideally annually, to reflect changes in exposure, vulnerability, and hazard characteristics. More frequent updates might be necessary after significant events or changes in building codes.
-
Q: Can PML be calculated for all types of risks? A: While PML is commonly used for natural catastrophes, it can also be applied to other types of risks, such as fire, terrorism, or pandemics. However, the specific calculation methods and data requirements will vary depending on the type of risk.
-
Q: What is the role of reinsurers in PML calculation? A: Reinsurers use PML calculations to assess the potential impact of catastrophic events on their portfolios and to design appropriate reinsurance programs. They often work closely with insurers to develop accurate PML estimates.
-
Q: How can I improve the accuracy of my PML calculations? A: Improving accuracy requires focusing on data quality. Ensure comprehensive exposure data, utilize high-resolution vulnerability assessments, and employ advanced catastrophe models with robust validation techniques. Consider using multiple modeling techniques for cross-validation.
-
Q: What are the implications of underestimating PML? A: Underestimating PML can lead to inadequate capital reserves, insufficient reinsurance coverage, and potentially severe financial consequences if a catastrophic event occurs.
Practical Tips
-
Invest in high-quality data: Accurate and comprehensive data is the foundation of reliable PML calculations.
-
Utilize advanced catastrophe models: These models incorporate complex interactions and provide more realistic estimates.
-
Conduct regular model validation: Compare model outputs with historical data to assess accuracy and identify potential biases.
-
Consider correlation of events: Account for the possibility of multiple events occurring simultaneously.
-
Incorporate uncertainty analysis: Quantify the uncertainty associated with PML estimates to provide a more complete picture of risk.
-
Integrate PML into a holistic risk management framework: Don't rely solely on PML; consider all relevant risk factors.
-
Consult with experts: Seek guidance from experienced actuaries, catastrophe modelers, and risk management professionals.
-
Stay updated on industry best practices: The field of risk modeling is constantly evolving; stay abreast of the latest advancements.
Final Conclusion
Probable Maximum Loss is a crucial concept for managing catastrophic risk. While its calculation involves complexity and relies on assumptions, the value of accurately estimating PML is undeniable. By employing sophisticated methodologies, high-quality data, and a holistic risk management approach, organizations can significantly improve their ability to prepare for and mitigate the impact of potentially devastating events. Further exploration into advanced modeling techniques, improved data collection methods, and better understanding of the uncertainties involved will continue to refine PML calculations and their role in safeguarding financial stability. The ongoing evolution of catastrophe modeling and risk management practices underscores the dynamic nature of this critical area, highlighting the need for continuous learning and adaptation.
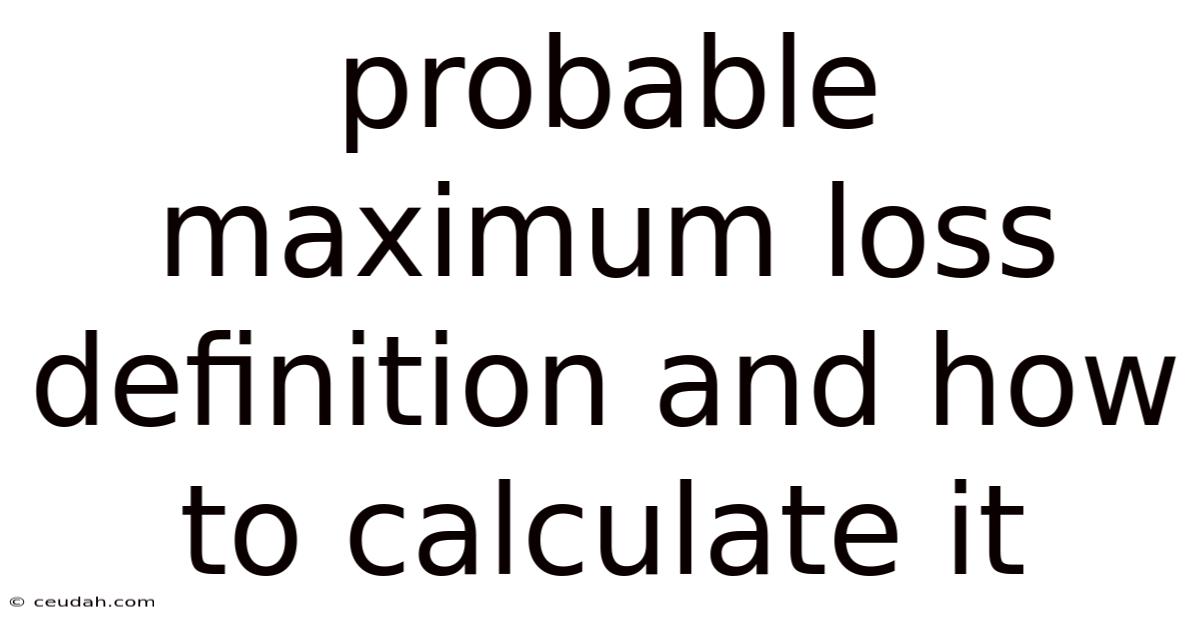
Thank you for visiting our website wich cover about Probable Maximum Loss Definition And How To Calculate It. We hope the information provided has been useful to you. Feel free to contact us if you have any questions or need further assistance. See you next time and dont miss to bookmark.
Also read the following articles
Article Title | Date |
---|---|
How Much Does Consumer Credit Counseling Service Cost | Apr 15, 2025 |
Purchase Money Security Interest Pmsi Definition How It Works | Apr 15, 2025 |
Price Persistence Definition | Apr 15, 2025 |
What Is Credit Spread | Apr 15, 2025 |
Fully Funded Documentary Letter Of Credit Ffdlc Definition | Apr 15, 2025 |